A new approach can train a machine learning model to predict the properties of a material using only data obtained through simple measurements, saving time and money compared with methods currently used.
The approach was designed by researchers at Japan’s National Institute for Materials Science, Asahi Kasei Corp., Mitsubishi Chemical Corp., Mitsui Chemicals Inc. and Sumitomo Chemical Co. Ltd. and reported in the journal “Science and Technology of Advanced Materials: Methods.”
“Machine learning is a powerful tool for predicting the composition of elements and process needed to fabricate a material with specific properties,” said Ryo Tamura, a senior researcher at NIMS who specializes in the field of materials informatics.
A tremendous amount of data usually is needed to train machine learning models for this purpose. Two kinds of data are used. Controllable descriptors are data that can be chosen without making a material, such as the chemical elements and processes used to synthesize it. But uncontrollable descriptors, like X-ray diffraction data, can be obtained only by making the material and conducting experiments on it.
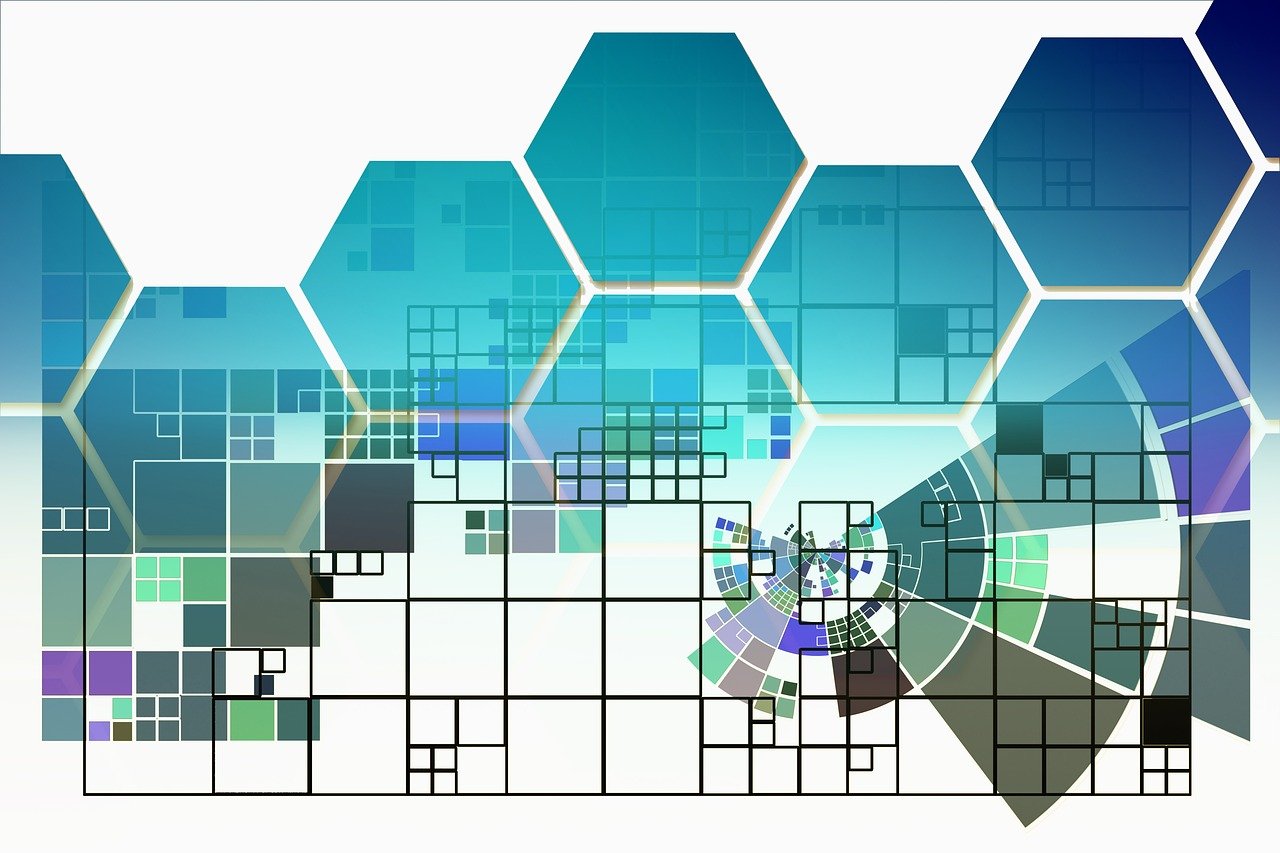
“We developed an effective experimental design method to more accurately predict material properties using descriptors that cannot be controlled,” Tamura said.
The approach involves the examination of a dataset of controllable descriptors to choose the best material with the target properties to use for improving the accuracy of the model. In this case, the scientists interrogated a database of 75 types of polypropylenes to select a candidate with specific mechanical properties. The researchers then selected the material and extracted some of its uncontrollable descriptors — for example, its X-ray diffraction data and mechanical properties. This data was added to the present dataset to better train a machine learning model employing special algorithms to predict material properties using only uncontrollable descriptors.
“Our experimental design can be used to predict difficult-to-measure experimental data using easy-to-measure data, accelerating our ability to design new materials or to repurpose already known ones while reducing the costs,” Tamura said.
The prediction method also can help improve understanding of how the structure of a material affects specific properties.
The team is working on further optimizing the approach in collaboration with chemical manufacturers in Japan.
For more information, contact Tamura at tamura.ryo@nims.go.jp.
— ResearchSEA/Asia Research News
Related Glossary Terms
- mechanical properties
mechanical properties
Properties of a material that reveal its elastic and inelastic behavior when force is applied, thereby indicating its suitability for mechanical applications; for example, modulus of elasticity, tensile strength, elongation, hardness and fatigue limit.