Contact Details
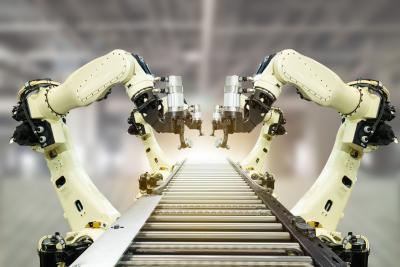
Speaking at the recent American Manufacturing Summit in Chicago, Frans Cronje, managing director and co-founder of DataProphet, a specialist in developing artificial intelligence (AI) solutions for the manufacturing sector, warned C-Suite executives from some of America’s largest companies that, with the unprecedented amount of data coming off the line, there is an urgent need to augment human analysis with AI, separating the data that can provide real value.
He commented: “Within the process control environment there is a huge amount of data coming off the line – too much for any one person to analyze. There is a real danger that operators are being blinded by a blizzard of information and are missing real opportunities for process and quality improvement. My message to manufacturers is that there is a large amount of value in your data and it does not require large capital investment to begin to extract it.”
He continued: “Manufacturing is complex and hard to control, and existing technologies often fail at preempting production glitches and fail to spot the changing conditions that can impact quality. Each process step can have hundreds of variables that affect the quality of the output and operational effectiveness. Even the skilled experts lose control of their process.”
Cronje said that with traditional statistical process control software, control limits are programmed by human experts and often fail to capture the complex interactions between all process variables. As a result, there is significant variability in plant performance and production quality that human experts cannot explain.
The solution, says Cronje, is to use AI to find complex patterns in datasets and develop distinct operating paradigms for the production facility. Crucially, modern AI systems, such as DataProphet’s OMNI product, an AI solution to reduce the cost of non-quality for heavy industries, which can then produce control plans that bring production into a stable and optimized state, developing a path from the current plant state to the optimal state.
He continued: “All of this is possible, using cutting-edge machine learning techniques, which allow OMNI to understand the effect that a small change in an arbitrary process step will have on all downstream process steps. Process steps are no longer operated and controlled in isolation and for each prescribed change, OMNI can quantify the expected magnitude of its effect on production."
Crucially, Cronje says that AI is delivering real ROI for heavy manufacturers. “We are seeing incredible results, such as a large foundry that has reduced internal and external scrap by more than $1 million per annum and an automotive bodyshop has reduced stud and spot-welding faults by more than 50 percent.”
Cronje also encouraged American manufacturers to learn from historical manufacturing data and not just assume that historical data has no value. “We need to learn from historical data, from PLCs and even old logbooks, to exploit the collective knowledge and experience of all those who have influenced the plant in the past. We need to institutionalize those years of operational experience for the benefit of the manufacturer’s current and future workforce – not lose them.”
Related Glossary Terms
- process control
process control
Method of monitoring a process. Relates to electronic hardware and instrumentation used in automated process control. See in-process gaging, inspection; SPC, statistical process control.
- statistical process control ( SPC)
statistical process control ( SPC)
Statistical techniques to measure and analyze the extent to which a process deviates from a set standard.